Modeling the Mechanism
Across science businesses we see the same challenges. Everyone wants winners faster and cheaper than they can test. If we could just perfect the process of "science," we could avoid getting stuck in the trap of waiting on unnecessary tests and phases.
Others have tried to solve this problem with "black box" AI/Machine Learning/statistical models which rely solely on passively observed data – they may even flaunt that they are "purely" data-driven and "uncontaminated" by the assumptions of theory or structure, but they only predict associations. Black Friday yields sales. Rain yields grain.
With recent advancements in the science of causality, we now know that we can do better. Our team of PhDs, computational scientists, MBAs, and applied mathematicians built a different modeling approach to predict outcomes under intervention. They tell you how to achieve goals you've never met by doing things you've never done. They work because they know why.
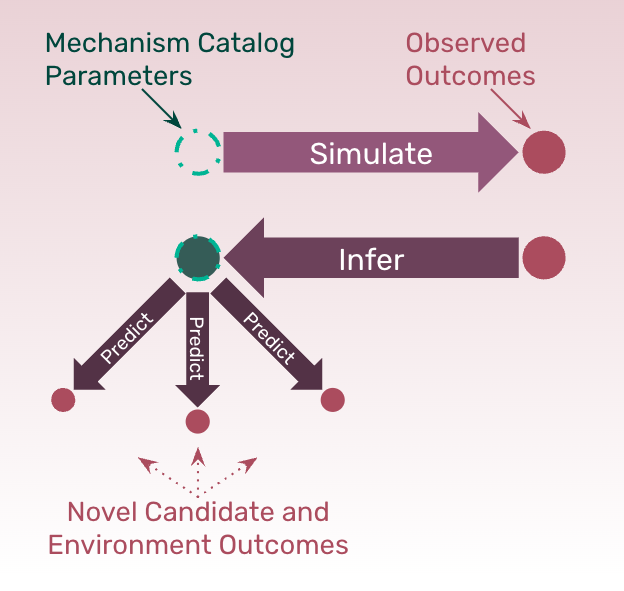
Build Data Richness
You struggle with enterprise systems because they cannot capture the richness of complex business and scientific processes. To get work done, subject matter experts have to preserve data crucial to understanding the product as tribal knowledge and ad-hoc pockets of data–outside of any corporate ERP, CRM, PLM, LIMS or ELN. Our solution enables quick integration from everywhere, yes even for that crucial data and metadata - getting past the roadblocks of ERP, etc.

Reveal Hidden Mechanisms
Your Design of Experiments is often focused on getting a specific outcome: "How do I improve this battery design's capacity?" or "How do I get a juicier tomato?" However, you run a disconnected series of experiments that don't reveal the "why". We disentangle, quantify, and structure unknown material properties (activity of an enzyme, diffusion through a crystal lattice, etc.) using our Bayesian Inverse technology and your existing testing data.
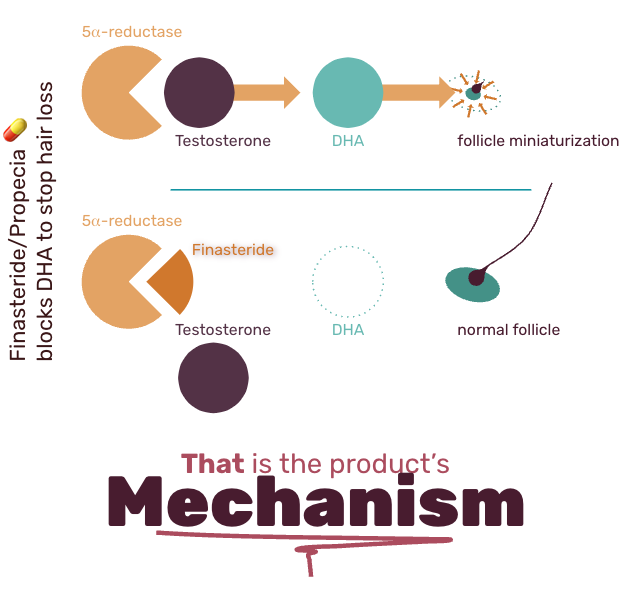
Capture Environmental Factors
Concurrent mechanisms aren't just entangled with each other - they're highly entangled with the environment. Research can't live in a vacuum, but scientific reductionism dissects mechanisms by excluding the richness of the environment. How do you know if your gene increased corn yields or was it just a wet year? Our environmental simulators recreate the experimental conditions of your physical tests and the complex real-world conditions of your products.

Technology Platform Components
"Super Suit" Architecture
Our platform's data architecture supports new data primitives for expressing the rich (nested, conditional and multimedia) structure of science-business data and a compact toolbox of new action primitives (like tree-aggregate and tree-compare) for accomplishing any complex workflow without app customization.
Universal Model Language
Our platform uses a form of Bayesian probabilistic programming as a universal language to describe any mechanistic simulation model. This allows us to weave mechanisms gathered from disparate academic literature sources into a singular whole.
Universal Parameter Learner
Our platform's Bayesian Inverse engine can learn–and learn the limits of its own knowledge–to parameterize the mechanisms of any mechanistic simulation model from your existing testing data without software or mathematical customizations.
Universal Business Optimizer
Our platform uses compiler technology and advanced mathematical methods to build environmental and business simulators that allow our software applications to translate mechanisms into optimized business decisions.